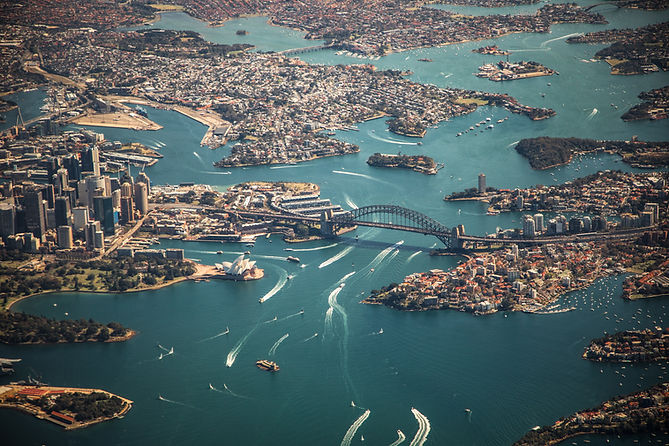
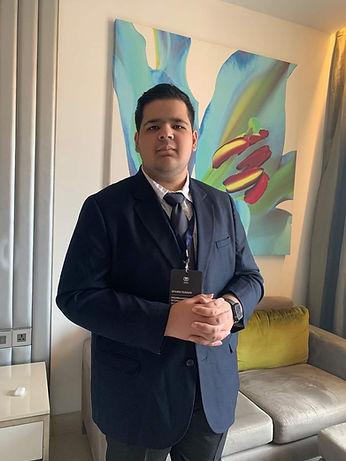
Sparsh Sumani
ML Enthusiast, Research Analyst & CS Student at UNSW
Phone:
(+61) 403 714 641
Email:
Address:
73 Albert Avenue, Chatswood (NSW 2067)
Sydney, New South Wales, Australia
Date of Birth:
April 7th, 2005
A Bit About Me
Hi, I’m Sparsh Sumani — a penultimate year Computer Science student at UNSW, specialising in Artificial Intelligence, and a Research Assistant working at the intersection of AI, data science, and real-world impact.
I’m deeply passionate about using neural networks and machine learning to solve complex problems — whether it’s optimising air travel routes for economic sustainability or building AI models that help retail investors make smarter trading decisions. My journey spans across aviation research, fintech innovation, and ethical AI, with hands-on experience in everything from building deep learning models for time-series forecasting to large-scale data analysis and startup development.
Outside the code, I’m deeply engaged in the world of finance and entrepreneurship — constantly exploring how AI can transform investing, trading, and the startup ecosystem. I enjoy building ventures from the ground up, designing fintech tools for emerging investors, and analyzing market behavior through the lens of data. When I’m not building or researching, you’ll often find me exploring new corners of Sydney, soaking in its culture, nature, and energy — always chasing fresh perspectives, whether through startups or the streets of the city.
Projects
Deep Learning for Air Route Viability Forecasting
UNSW
Designed and trained multi-layer Artificial Neural Networks (ANNs) and GRU-based Recurrent Neural Networks (RNNs) to forecast monthly passenger volumes and fare pricing for a proposed Tokyo Narita (NRT) – Uluru (AYQ) international route.
Implemented advanced feature engineering (cyclical temporal encoding, Haversine distance, GDP indicators) and leveraged sequence modeling for seasonal pattern capture.
Models achieved >93% predictive accuracy (ANN R² = 0.95, GRU R² = 0.92), estimating monthly demand between 1500–1800 passengers and validating route viability with projected $2.25M annual revenue.
RNN-Based Delay Forecasting for JetBlue
UNSW
Engineered LSTM and GRU-based neural networks to forecast JetBlue’s operational delays using sequential OTP data at both daily and hourly resolution.
Designed multivariate time-series models with cyclical temporal encodings, lagged delay features, and hourly disruption metrics, enabling both regression and classification pipelines.
Built a deep GRU classifier for short-term delay threshold detection, achieving high precision and recall in identifying operational risk periods, with regression models capturing intra-day delay trends at MAE < 0.09.